1. |
Pióro M., Wołczyk M.♦, Pascanu R.♦, Von Oswald J.♦, Sacramento J.♦, State soup: in-context skill learning, retrieval and mixing,
Next Generation of Sequence Modeling Architectures Workshop at International Conference on Machine Learning 2024, 2024-07-26/07-26, Wiedeń (AT), pp.1-4, 2024 Streszczenie: A new breed of gated-linear recurrent neural networks has reached state-of-the-art performance on a range of sequence modeling problems. Such models naturally handle long sequences efficiently, as the cost of processing a new input is independent of sequence length. Here, we explore another advantage of these stateful sequence models, inspired by the success of model merging through parameter interpolation. Building on parallels between fine-tuning and in-context learning, we investigate whether we can treat internal states as task vectors that can be stored, retrieved, and then linearly combined, exploiting the linearity of recurrence. We study this form of fast model merging on Mamba-2.8b, a pretrained recurrent model, and present preliminary evidence that simple linear state interpolation methods suffice to improve next-token perplexity as well as downstream in-context learning task performance. Afiliacje autorów:
Pióro M. | - | IPPT PAN | Wołczyk M. | - | inna afiliacja | Pascanu R. | - | inna afiliacja | Von Oswald J. | - | inna afiliacja | Sacramento J. | - | inna afiliacja |
| 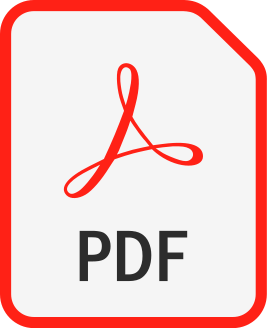 |