1. |
Pawłowska A., Żołek N., Preliminary Feature Extraction for Small Lesion Classification in Sonomammographic Images,
UFFC-JS, 2024 IEEE Ultrasonics, Ferroelectrics, and Frequency Control Joint Symposium, 2024-09-22/09-26, Tajpej (TW), pp.1-4, 2024 Streszczenie: In breast cancer diagnosis, early detection of tumors and accurate differentiation of malignant and benign breast lesions are key demands. Tumor size, as a measure of tumor progression, is related to recurrence rate and patient survival. This study aims to determine which sonographic features al- low the differentiation of small breast lesions into benign and malignant. Inclusion criteria for the analysis were tumors with the longest diameter of less than or equal to 10 mm and tumors with confirmed classification by follow-up care or core needle biopsy result. Following the criteria, 1515 cases were analyzed, including 365 carcinomas and 1150 benign lesions. To quantitatively evaluate the images, 383 ultrasound parameters
(BI-RADS features, morphological features, fractal features, his- togram features, and texture parameters) were used. Univariate and multiple logistic regression analyses were used to assess the significance of various diagnostic features and their combinations. The combined classifier (based on 19 quantitative features) yields an area under the ROC curve of 0.91. Słowa kluczowe: Ultrasound imaging, Breast cancer, Small lesion, Feature extraction Afiliacje autorów:
Pawłowska A. | - | IPPT PAN | Żołek N. | - | IPPT PAN |
| 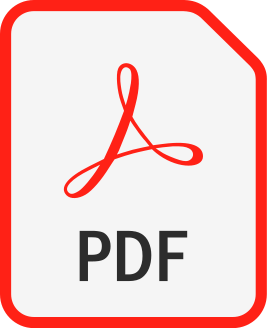 |
2. |
Fura Ł., Pawłowska A., Ćwierz-Pieńkowska A.♦, Domalik A.♦, Jaguś D.♦, Kasprzak P.♦, Matkowski R.♦, Żołek N., Analysis of uncertainty in accuracy of the reference segmentation of ultrasound images of breast tumors,
SPIE Medical Imaging 2024, 2024-02-18/02-22, San Diego (US), DOI: 10.1117/12.3006442, pp.1-5, 2024 Streszczenie: Manual image segmentations are naturally subject to inaccuracies related to systematic errors (due to the tools used, eye-hand coordination, etc.). This was noted earlier when a simplified accuracy scale was proposed [1]. This scale arbitrarily divides a given range of values of the Kappa measurement parameter into classes: almost perfect (>0.80), substantial (0.61 - 0.80), moderate (0.41 - 0.60), fair (0.21 - 0.40), slight (0.00 - 0.21) and poor (< 0.00). However, the determination of threshold values between classes is not entirely clear and seems to be application-dependent. This is particularly important for images in which the tumor-normal tissue boundary can be very indistinct, as is observed in ultrasound imaging of the most common cancer in women - breast cancer [2]. In machine learning, there is an ongoing contest over the values of performance indicators obtained from new neural network architecture without accounting for any ground truth bias. This raises the question of what relevance, from a segmentation quality point of view, a gain at the level of single percentages has [3] if the references have much greater uncertainty. So far, research on this topic has been limited. The relationship between the segmentations of breast tumors on ultrasound images provided by three radiologists and those obtained using deep learning model has been studied in [4]. Unfortunately, the indicated segmentation contour sometimes varied widely in all three cases. A cursory analysis by multiple physicians, which focused only on the Kappa coefficient in the context of physicians’ BI-RADS category assignments, was conducted in the [5]. In this article, we present a preliminary analysis of the accuracy of experts’ manually prepared binary breast cancer masks on ultrasound images and their impact on performance metrics commonly used in machine learning. In addition, we examined how tumor type or BI-RADS category [6] affects the accuracy of tumor contouring. Afiliacje autorów:
Fura Ł. | - | IPPT PAN | Pawłowska A. | - | IPPT PAN | Ćwierz-Pieńkowska A. | - | inna afiliacja | Domalik A. | - | inna afiliacja | Jaguś D. | - | inna afiliacja | Kasprzak P. | - | inna afiliacja | Matkowski R. | - | inna afiliacja | Żołek N. | - | IPPT PAN |
| 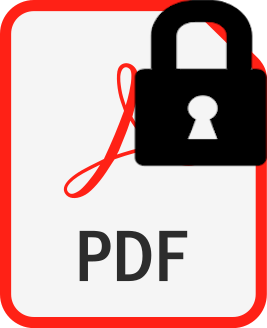 |
3. |
Pawłowska A., Żołek N., Litniewski J., Simulations of acoustic wave propagation in the breast with tumors using a modified VICTRE phantom,
IUS 2022, IEEE, International Ultrasonic Symposium, 2022-10-10/10-13, Wenecja (IT), DOI: 10.1109/IUS54386.2022.9958723, pp.1-4, 2022 Streszczenie: Understanding the relationship between acoustic properties of breast lesions and resulting ultrasound images may contribute to an earlier and more accurate diagnosis of the most common cancer in women. In addition to in vitro studies, in silico tumor models can provide a lot of crucial information due to the possibility of precise determination of the influence of changes in tissue structure on the resulting ultrasound echoes. The purpose was to develop the numerical phantom of the breast with the tumor for a reliable simulation of ultrasound images. In modeling the tissue structures of the breast, the VICTRE phantom, developed by the FDA for the simulation of X-ray mammography, was used. The numerical ultrasound model of breast cancer allows the simulation of ultrasound signals and images. It could be used to interpret, validate and develop new ultrasound methods for cancer diagnosis Słowa kluczowe: breast tumor, numerical phantom, ultrasound imaging Afiliacje autorów:
Pawłowska A. | - | IPPT PAN | Żołek N. | - | IPPT PAN | Litniewski J. | - | IPPT PAN |
| 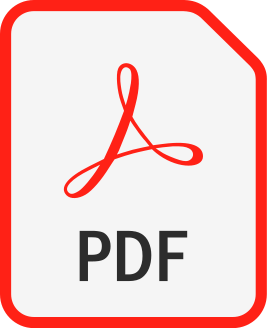 | 20p. |
4. |
Byra M., Karwat P., Ryzhankow I., Komorowski P.♦, Klimonda Z., Fura Ł., Pawłowska A., Żołek N., Litniewski J., Deep meta-learning for the selection of accurate ultrasound based breast mass classifier,
IUS 2022, IEEE, International Ultrasonic Symposium, 2022-10-10/10-13, Wenecja (IT), DOI: 10.1109/IUS54386.2022.9957191, pp.1-4, 2022 Streszczenie: Standard classification methods based on hand-crafted morphological and texture features have achieved good performance in breast mass differentiation in ultrasound (US).
In comparison to deep neural networks, commonly perceived as ‘black-box’ models, classical techniques are based on features that have well-understood medical and physical interpretation. However, classifiers based on morphological features commonly
underperform in the presence of the shadowing artifact and ill-defined mass borders, while texture based classifiers may fail when the US image is too noisy. Therefore, in practice it would be beneficial to select the classification method based on the appearance of the particular US image. In this work, we develop a deep meta-network that can automatically process input breast mass US images and recommend whether to apply the shape or
texture based classifier for the breast mass differentiation. Our preliminary results demonstrate that meta-learning techniques can be used to improve the performance of the standard classifiers based on handcrafted features. With the proposed meta-learning based approach, we achieved the area under the receiver operating characteristic curve of 0.95 and accuracy of 0.91.
Słowa kluczowe: breast mass classification, deep learning, meta-learning, morphological features, texture features Afiliacje autorów:
Byra M. | - | IPPT PAN | Karwat P. | - | IPPT PAN | Ryzhankow I. | - | IPPT PAN | Komorowski P. | - | inna afiliacja | Klimonda Z. | - | IPPT PAN | Fura Ł. | - | IPPT PAN | Pawłowska A. | - | IPPT PAN | Żołek N. | - | IPPT PAN | Litniewski J. | - | IPPT PAN |
| 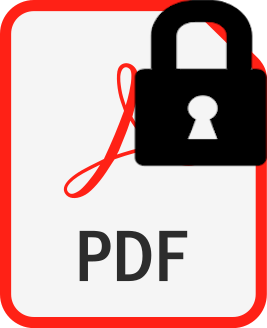 | 20p. |