1. |
Marszałek A.♦, Burczyński T., Modeling of limit order book data with ordered fuzzy numbers,
APPLIED SOFT COMPUTING, ISSN: 1568-4946, DOI: 10.1016/j.asoc.2024.111555, Vol.158, pp.1-20, 2024 Streszczenie: This paper presents a novel approach to representing the Limit Order Book data at a given timestamp using the Ordered Fuzzy Numbers concept. The limit order book contains all buy and sell orders placed by investors, updated in real-time, for the most liquid securities, even several hundred times a minute. Due to its irregular nature (different and dynamic changes in the number of buy and sell orders), direct calculations on the order book data are not feasible without transforming it into feature vectors. Currently, most studies use a price level-based data representation scheme when applying deep learning models on limit order book data. However, this scheme has limitations, particularly its sensitivity to subtle perturbations that can negatively impact model performance. On the other hand, the ordered fuzzy number is a mathematical object (a pair of two functions) used to process imprecise and uncertain data. Ordered Fuzzy Numbers possess well-defined arithmetic properties. Converting the limit order book data to ordered fuzzy numbers allows the creation of a time series of ordered fuzzy numbers (order books) and use them for further calculations, e.g., to represent input data for deep learning models or employing the concept of fuzzy time series in various domains, such as defining liquidity measures based on limit order book data. In this paper, the proposed approach is tested using one-year market data from the Polish Stock Exchange for the five biggest companies. The DeepLOB model is employed to predict mid-price movement using different input data representations. The proposed representation of Limit Order Book data demonstrated remarkably stable out-of-sample prediction accuracy, even when subjected to data perturbation. Słowa kluczowe: Limit order book, Ordered fuzzy number, High-frequency forecasting, Mid-price, Data perturbation Afiliacje autorów:
Marszałek A. | - | Cracow University of Technology (PL) | Burczyński T. | - | IPPT PAN |
| 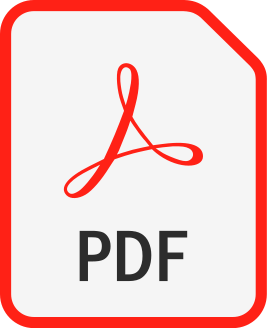 | 200p. |
2. |
Marszałek A.♦, Burczyński T., Forecasting day-ahead spot electricity prices using deep neural networks with attention mechanism,
Journal of Smart Environments and Green Computing, ISSN: 2767-6595, DOI: 10.20517/jsegc.2021.02, Vol.1, pp.21-31, 2021 Streszczenie: This paper presents a novel approach to forecast hourly day-ahead electricity prices. In recent years, many predictive models based on statistical methods and machine learning (deep learning) techniques have been proposed. However, the approach presented in this paper focuses on the problem of constructing a fair and unbiased model. In this considered case, unbiased means that the model can increase prediction accuracy and decrease categorical bias across different data clusters. For this purpose, a model combining techniques such as long short-term memory (LSTM) recurrent neural network, attention mechanism, and clustering is created. The proposed model’s main feature is that the attention weights for LSTM hidden states are calculated considering a context vector given for each sample individually as the cluster center to which the sample belongs. In training mode, the samples are iteratively (one time per epoch) clustered based on representation vectors given by the attention mechanism. In the empirical study, the proposed model was applied and evaluated on the Nord Pool market data. To confirm that the model decreases categorical bias, the obtained results were compared with results of similar LSTM models but without the proposed attention mechanism. Słowa kluczowe: deep learning, electricity prices forecasting, time series forecasting, attention mechanism, debiasing, Nord Pool data Afiliacje autorów:
Marszałek A. | - | Cracow University of Technology (PL) | Burczyński T. | - | IPPT PAN |
| 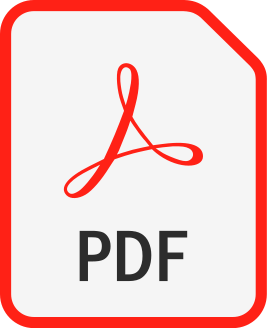 |
3. |
Marszałek A.♦, Burczyński T., Ordered fuzzy random variables: Definition and the concept of normality,
INFORMATION SCIENCES, ISSN: 0020-0255, DOI: 10.1016/j.ins.2020.08.120, pp.1-12, 2020 Streszczenie: The concept of fuzzy random variable combines two sources of uncertainty: randomness and fuzziness, whereas the model of ordered fuzzy numbers provides a representation of inaccurate quantitative data, and is an alternative to the standard fuzzy numbers model proposed by Zadeh. This paper develops the model of ordered fuzzy numbers by defining the concept of fuzzy random variables for these numbers, called further ordered fuzzy random variables. Thanks to the well-defined arithmetic of ordered fuzzy numbers (existence of neutral and opposite elements) and the introduced ordered fuzzy random variables; it becomes possible to construct fully fuzzy stochastic time series models such as e.g., the autoregressive model or the GARCH model in the form of classical equations, which can be estimated using the least-squares or the maximum likelihood method. Furthermore, the concept of normality of ordered fuzzy random variables and the method to generate pseudo-random ordered fuzzy variables with normal distribution are introduced. Słowa kluczowe: ordered fuzzy numbers, fuzzy random variables, ordered fuzzy random variables, normal ordered fuzzy random variable Afiliacje autorów:
Marszałek A. | - | Cracow University of Technology (PL) | Burczyński T. | - | IPPT PAN |
| 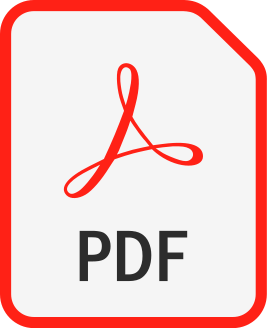 | 200p. |
4. |
Marszałek A.♦, Burczyński T., Modeling and forecasting financial time series with ordered fuzzy candlesticks,
INFORMATION SCIENCES, ISSN: 0020-0255, DOI: 10.1016/j.ins.2014.03.026, Vol.273, pp.144-155, 2014 Streszczenie: The goal of the paper is to present an experimental evaluation of fuzzy time series models which are based on ordered fuzzy numbers to predict financial time series. Considering this approach the financial data is modeled using Ordered Fuzzy Numbers (OFNs) called further by Ordered Fuzzy Candlesticks (OFCs). The use of them allows modeling uncertainty associated with financial data and maintaining more information about price movement at assumed time interval than comparing to commonly used price charts (e.g. Japanese Candlestick chart). Thanks to well-defined arithmetic of OFN, one can construct models of fuzzy time series, such as an Ordered Fuzzy Autoregressive Process (OFAR), where all input values are OFC, while the coefficients and output values are arbitrary OFN; in the form of classical equations, without using rule-based systems. In an empirical study ordered fuzzy autoregressive models are applied to modeling and predict price movement of futures contracts on Warsaw Stock Exchange Top 20 Index. Słowa kluczowe: Ordered fuzzy number, Directional predictability, Fuzzy autoregressive, Financial time series, Stock return Afiliacje autorów:
Marszałek A. | - | Cracow University of Technology (PL) | Burczyński T. | - | IPPT PAN |
| 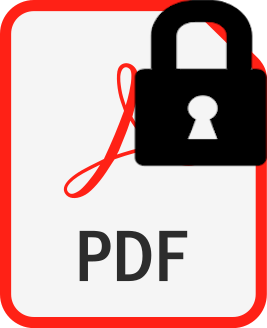 | 45p. |
5. |
Marszałek A.♦, Burczyński T.♦, Financial Fuzzy Time Series Models Based on Ordered Fuzzy Numbers,
Intelligent Systems Reference Library, ISSN: 1868-4394, DOI: 10.1007/978-3-642-33439-9_4, Vol.47, pp.77-95, 2013 Streszczenie: The purpose of this chapter is to present an original concept of financial fuzzy time series models based on financial data in the form of Japanese Candlestick Charts. In this approach the Japanese Candlesticks are modeled using Ordered Fuzzy Numbers (OFN) called further Ordered Fuzzy Candlesticks (OFC). The use of ordered fuzzy numbers allows modeling uncertainty associated with financial data. Thanks to well-defined arithmetic of ordered fuzzy numbers, one can construct models of fuzzy time series, such as e.g. an autoregressive process, where all input values are OFC, while the coefficients and output values are arbitrary OFN, in the form of classical equations, without using rule-based systems. Finally, several applications of these models for modeling and forecasting selected financial time series are presented. Słowa kluczowe: Fuzzy Number, Arithmetic Operation, Autoregressive Process Afiliacje autorów:
Marszałek A. | - | Cracow University of Technology (PL) | Burczyński T. | - | inna afiliacja |
| 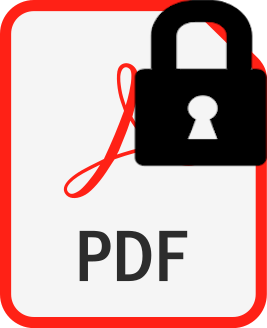 |