1. |
Badora M., Bartosik P.♦, Graziano A.♦, Szolc T.A., Using physics-informed neural networks with small datasets to predict the length of gas turbine nozzle cracks,
Advanced Engineering Informatics, ISSN: 1474-0346, DOI: 10.1016/j.aei.2023.102232, Vol.58, No.102232, pp.1-16, 2023 Abstract: We created a Physics-Informed Neural Network (PINN) to model the propagation of fatigue cracks. The analyzed object is a high-pressure Nozzle of an industrial gas turbine. The models are based on a Recurrent Neural Network with an embedded Feedforward Neural Network to estimate the stress intensity factor. The thermal stresses are calculated based on engine operational data, leveraging a Finite Element Analysis. However, the time series are available just for 54% of the start-stop cycles, and only 13 crack measures were recorded. Three separate models were trained based on ten, two, and one observation, respectively. The importance of the empirical data was regulated during the training to avoid solutions inconsistent with the underlying physics. The models generalize well and predict accurately also outside the training domain. Additionally, we propose a novel method of scaling models based on PINNs and transferring knowledge between domains. It enables predicting in the target domain, even if damage measures are unavailable. The obtained results confirm the effectiveness of this approach. Keywords: Physics-informed neural networks , Predictive maintenance , Fatigue cracks , Regression analysis , Small data , Turbomachinery Affiliations:
Badora M. | - | IPPT PAN | Bartosik P. | - | other affiliation | Graziano A. | - | other affiliation | Szolc T.A. | - | IPPT PAN |
| 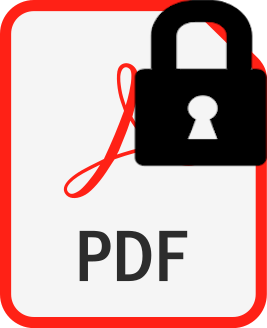 |