1. |
Deshpande S.♦, Rappel H.♦, Hobbs M.♦, Bordas S.♦, Lengiewicz J.A., Gaussian process regression + deep neural network autoencoder for probabilistic surrogate modeling in nonlinear mechanics of solids,
COMPUTER METHODS IN APPLIED MECHANICS AND ENGINEERING, ISSN: 0045-7825, DOI: 10.1016/j.cma.2025.117790, Vol.437, No.117790, pp.1-17, 2025 Abstract: Many real-world applications demand accurate and fast predictions, as well as reliable uncertainty estimates. However, quantifying uncertainty on high-dimensional predictions is still a severely under-investigated problem, especially when input–output relationships are non-linear. To handle this problem, the present work introduces an innovative approach that combines autoencoder deep neural networks with the probabilistic regression capabilities of Gaussian processes. The autoencoder provides a low-dimensional representation of the solution space, while the Gaussian process is a Bayesian method that provides a probabilistic mapping between the low-dimensional inputs and outputs. We validate the proposed framework for its application to surrogate modeling of non-linear finite element simulations. Our findings highlight that the proposed framework is computationally efficient as well as accurate in predicting non-linear deformations of solid bodies subjected to external forces, all the while providing insightful uncertainty assessments. Keywords: Surrogate modeling,Deep neural networks,Gaussian proces,Autoencoders,Uncertainty quantification,Finite element method Affiliations:
Deshpande S. | - | University of Luxembourg (LU) | Rappel H. | - | other affiliation | Hobbs M. | - | other affiliation | Bordas S. | - | other affiliation | Lengiewicz J.A. | - | IPPT PAN |
| 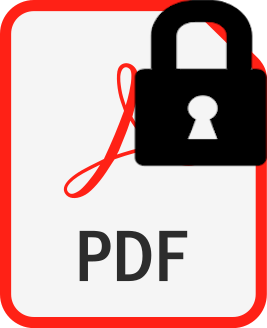 |
2. |
Shen Z.♦, Sosa R.♦, Bordas S.♦, Tkatchenko A.♦, Lengiewicz J. A., Quantum-informed simulations for mechanics of materials: DFTB+MBD framework,
International Journal of Engineering Science, ISSN: 0020-7225, DOI: 10.1016/j.ijengsci.2024.104126, Vol.204, No.104126, pp.1-18, 2024 Abstract: The macroscopic behaviors of materials are determined by interactions that occur at multiple lengths and time scales. Depending on the application, describing, predicting, and understanding these behaviors may require models that rely on insights from atomic and electronic scales. In such cases, classical simplified approximations at those scales are insufficient, and quantum-based modeling is required. In this paper, we study how quantum effects can modify the mechanical properties of systems relevant to materials engineering. We base our study on a high-fidelity modeling framework that combines two computationally efficient models rooted in quantum first principles: Density Functional Tight Binding (DFTB) and many-body dispersion (MBD). The MBD model is applied to accurately describe non-covalent van der Waals interactions. Through various benchmark applications, we demonstrate the capabilities of this framework and the limitations of simplified modeling. We provide an open-source repository containing all codes, datasets, and examples presented in this work. This repository serves as a practical toolkit that we hope will support the development of future research in effective large-scale and multiscale modeling with quantum-mechanical fidelity. Keywords: DFT, DFTB, Energy range separation, Many-body dispersion, van der Waals interaction, Carbon nanotube, UHMWPE Affiliations:
Shen Z. | - | other affiliation | Sosa R. | - | other affiliation | Bordas S. | - | other affiliation | Tkatchenko A. | - | other affiliation | Lengiewicz J. A. | - | IPPT PAN |
| 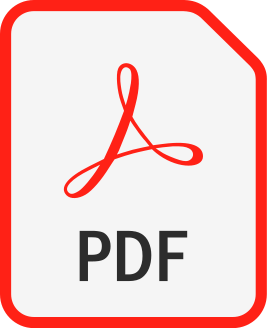 |
3. |
Deshpande S.♦, Bordas S.♦, Lengiewicz J. A., MAgNET: A graph U-Net architecture for mesh-based simulations,
Engineering Applications of Artificial Intelligence, ISSN: 0952-1976, DOI: 10.1016/j.engappai.2024.108055, Vol.133 B, No.108055, pp.1-18, 2024 Abstract: In many cutting-edge applications, high-fidelity computational models prove to be too slow for practical use and are therefore replaced by much faster surrogate models. Recently, deep learning techniques have increasingly been utilized to accelerate such predictions. To enable learning on large-dimensional and complex data, specific neural network architectures have been developed, including convolutional and graph neural networks. In this work, we present a novel encoder–decoder geometric deep learning framework called MAgNET, which extends the well-known convolutional neural networks to accommodate arbitrary graph-structured data. MAgNET consists of innovative Multichannel Aggregation (MAg) layers and graph pooling/unpooling layers, forming a graph U-Net architecture that is analogous to convolutional U-Nets. We demonstrate the predictive capabilities of MAgNET in surrogate modeling for non-linear finite element simulations in the mechanics of solids. Keywords: Geometric deep learning, Mesh based simulations, Finite element method, Graph U-Net, Surrogate modeling Affiliations:
Deshpande S. | - | University of Luxembourg (LU) | Bordas S. | - | other affiliation | Lengiewicz J. A. | - | IPPT PAN |
| 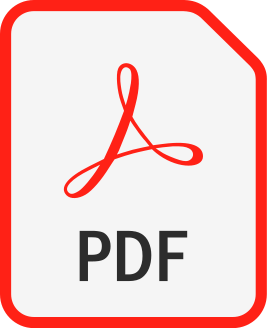 |