1. |
Poon Ch.♦, Byra M., Shimogori T.♦, Skibbe H.♦, Meta-Learning for Segmentation of In Situ Hybridization Gene Expression Images,
MIDL Paris 2024, Medical Imaging with Deep Learning, 2024-07-03/07-05, Paryż (FR), No.031, pp.1-4, 2024 Abstract: Segmentation of biomedical images is often ambiguous and complicated by noise, varying
contrasts, and imaging artifacts. We address the challenge of segmenting images of brain
tissue in which gene expression has been localized using in situ hybridization. Since gene
expression patterns differ widely between genes, it can be difficult to correctly discriminate
pixels positive for gene expression. In testing different segmentation networks, we observed
that each network had its own trade-offs between sensitivity and precision. To exploit
the benefits of all trained networks, we developed a meta-network that learns to combine
multiple segmentation maps from diverse segmentation architectures to generate a final
segmentation that best matches the ground-truth label. In our experiments, the meta-
network outperforms ensembles that simply average segmentation maps. Keywords: meta-learning, segmentation, gene expression Affiliations:
Poon Ch. | - | other affiliation | Byra M. | - | IPPT PAN | Shimogori T. | - | other affiliation | Skibbe H. | - | other affiliation |
| 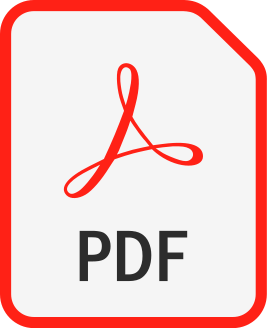 |
2. |
Poon Ch.♦, Rachmadi M.F.♦, Byra M., Schlachter M.♦, Xu B.♦, Shimogori T.♦, Skibbe H.♦, AN AUTOMATED PIPELINE TO CREATE AN ATLAS OF IN SITU HYBRIDIZATION GENE EXPRESSION DATA IN THE ADULT MARMOSET BRAIN,
ISBI, 2023 IEEE 20th International Symposium on Biomedical Imaging, 2023-04-18/04-21, Cartagena (CO), DOI: 10.1109/ISBI53787.2023.10230544, pp.1-5, 2023 Abstract: We present the first automated pipeline to create an atlas of in situ hybridization gene expression in the adult marmoset brain in the same stereotaxic space. The pipeline consists of segmentation of gene expression from microscopy images and registration of images to a standard space. Automation of this pipeline is necessary to analyze the large volume of data in the genome-wide whole-brain dataset, and to process images that have varying intensity profiles and expression patterns with minimal human bias. To reduce the number of labelled images required for training, we develop a semi-supervised segmentation model. We further develop an iterative algorithm to register images to a standard space, enabling comparative analysis between genes and concurrent visualization with other datasets, thereby facilitating a more holistic understanding of primate brain structure and function. Keywords: contrastive learning, gene atlas, segmen-tation, semi-supervised learning, registration Affiliations:
Poon Ch. | - | other affiliation | Rachmadi M.F. | - | other affiliation | Byra M. | - | IPPT PAN | Schlachter M. | - | other affiliation | Xu B. | - | Tsinghua University (CN) | Shimogori T. | - | other affiliation | Skibbe H. | - | other affiliation |
| 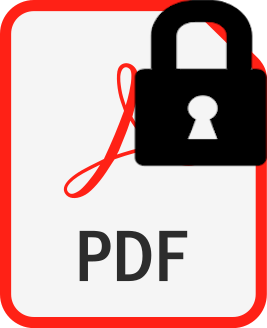 |
3. |
Byra M., Poon Ch.♦, Shimogori T.♦, Skibbe H.♦, Implicit Neural Representations for Joint Decomposition and Registration of Gene Expression Images in the Marmoset Brain,
MICCAI 2023, Medical Image Computing and Computer-Assisted Intervention, 2023-10-08/10-12, Vancouver (CA), DOI: 10.48550/arXiv.2308.04039, pp.1, 2023 Abstract: We propose a novel image registration method based on implicit neural representations that addresses the challenging problem of registering a pair of brain images with similar anatomical structures, but where one image contains additional features or artifacts that are not present in the other image. To demonstrate its effectiveness, we use 2D microscopy in situ hybridization gene expression images of the marmoset brain. Accurately quantifying gene expression requires image registration to a brain template, which is difficult due to the diversity of patterns causing variations in visible anatomical brain structures. Our approach uses implicit networks in combination with an image exclusion loss to jointly perform the registration and decompose the image into a support and residual image. The support image aligns well with the template, while the residual image captures individual image characteristics that diverge from the template. In experiments, our method provided excellent results and outperformed other registration techniques. Keywords: brain, deep learning, gene expression, implicit neural representations, registration Affiliations:
Byra M. | - | IPPT PAN | Poon Ch. | - | other affiliation | Shimogori T. | - | other affiliation | Skibbe H. | - | other affiliation |
| 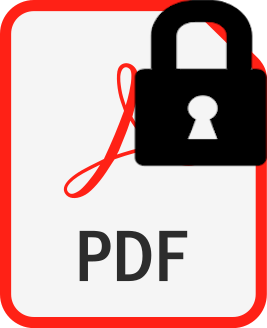 |