1. |
Byra M., Styczyński G.♦, Szmigielski C.♦, Kalinowski P.♦, Michałowski Ł.♦, Paluszkiewicz R.♦, Ziarkiewicz-Wróblewska B.♦, Zieniewicz K.♦, Sobieraj P.♦, Nowicki A., Transfer learning with deep convolutiona lneural network for liver steatosis assessment in ultrasound images,
International Journal of Computer Assisted Radiology and Surgery, ISSN: 1861-6410, DOI: 10.1007/s11548-018-1843-2, Vol.13, No.12, pp.1895-1903, 2018 Abstract: Purpose
The nonalcoholic fatty liver disease is the most common liver abnormality. Up to date, liver biopsy is the reference standard for direct liver steatosis quantification in hepatic tissue samples. In this paper we propose a neural network-based approach for nonalcoholic fatty liver disease assessment in ultrasound.
Methods
We used the Inception-ResNet-v2 deep convolutional neural network pre-trained on the ImageNet dataset to extract high-level features in liver B-mode ultrasound image sequences. The steatosis level of each liver was graded by wedge biopsy. The proposed approach was compared with the hepatorenal index technique and the gray-level co-occurrence matrix algorithm. After the feature extraction, we applied the support vector machine algorithm to classify images containing fatty liver. Based on liver biopsy, the fatty liver was defined to have more than 5% of hepatocytes with steatosis. Next, we used the features and the Lasso regression method to assess the steatosis level.
Results
The area under the receiver operating characteristics curve obtained using the proposed approach was equal to 0.977, being higher than the one obtained with the hepatorenal index method, 0.959, and much higher than in the case of the gray-level co-occurrence matrix algorithm, 0.893. For regression the Spearman correlation coefficients between the steatosis level and the proposed approach, the hepatorenal index and the gray-level co-occurrence matrix algorithm were equal to 0.78, 0.80 and 0.39, respectively.
Conclusions
The proposed approach may help the sonographers automatically diagnose the amount of fat in the liver. The presented approach is efficient and in comparison with other methods does not require the sonographers to select the region of interest. Keywords: Nonalcoholic fatty, liver disease, Ultrasound imaging Deep learning, Convolutional neural networks, Hepatorenal index, Transfer learning Affiliations:
Byra M. | - | IPPT PAN | Styczyński G. | - | Medical University of Warsaw (PL) | Szmigielski C. | - | Medical University of Warsaw (PL) | Kalinowski P. | - | Medical University of Warsaw (PL) | Michałowski Ł. | - | Medical University of Warsaw (PL) | Paluszkiewicz R. | - | Medical University of Warsaw (PL) | Ziarkiewicz-Wróblewska B. | - | Medical University of Warsaw (PL) | Zieniewicz K. | - | Medical University of Warsaw (PL) | Sobieraj P. | - | Medical University of Warsaw (PL) | Nowicki A. | - | IPPT PAN |
| 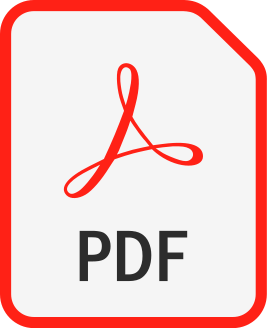 |