1. |
Jedlińska A., Pisarski D., Mikułowski G., Błachowski B., Jankowski Ł., Damage detection in a semi-active structural control system based on reinforcement learning,
ISMA 2024, 31st International Conference on Noise and Vibration Engineering, 2024-09-09/09-11, Leuven (BE), pp.1-9, 2024 Abstract: This contribution applies the machine learning technique of reinforcement learning for simultaneous damage detection and control of structures. The proposed system consists of two components. The control component is responsible for semi-active mitigation of vibrations. The control law is determined experimentally in a trial-and-error interaction with a simulated environment. The process is data-driven: the control agent iteratively improves its control law based on the observed results of past control actions. The robustness relies on the accuracy of the structural model used for training. The control efficiency can decrease if the physical structure is damaged and diverges from the model, that is, when effective control may be most required. Thus, the second component of the proposed system monitors the structure to detect damages and inform the control component. The approach is tested in a numerical experiment of a shear-building under random seismic-type excitation. A semi-active tuned mass damper (TMD) is used as an actuator, and a classical TMD serves as a reference. Keywords: semi-active control, structural control, structural monitoring, reinforcement learning, machine learning Affiliations:
Jedlińska A. | - | IPPT PAN | Pisarski D. | - | IPPT PAN | Mikułowski G. | - | IPPT PAN | Błachowski B. | - | IPPT PAN | Jankowski Ł. | - | IPPT PAN |
| 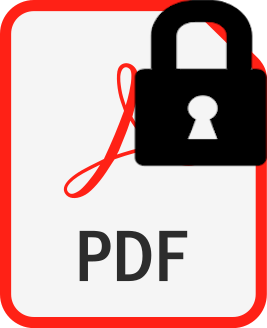 |
2. |
Jedlińska A., Pisarski D., Mikułowski G., Błachowski B., Jankowski Ł., Damage-aware structural control based on reinforcement learning,
WEWSHM 2024, 11th European Workshop on Structural Health Monitoring, 2024-06-10/06-13, Potsdam (DE), DOI: doi.org/10.58286/29606, pp.1-8, 2024 Abstract: This contribution presents a semi-active control technique intended for mitigation of structural vibrations. The control law is derived in a repeated trial-and-error interaction between the control agent and a simulated environment. The experience-based training approach is used which is the defining feature of the machine learning techniques of reinforcement learning (RL), implemented here using the framework provided by Deep Q Learning (DQN). The involved artificial neural network not only determines the control action, but additionally identifies structural damages, which is a nontrivial task due to the nonlinearity of the control. This requires a specific multi-head architecture, which allows the network to be damage-aware, and a specific training procedure, where the memory pool preserved for the RL stage of experience replay is populated with not only the observations, control actions, and rewards, but also with the momentary status of structural damage. Such an approach can be used to explicitly promote the damage-awareness of the control agent. The proposed technique is tested and verified in a numerical example of a shear-type building model subjected to a random seismic-type excitation. A tuned mass damper (TMD) with a controllable level of viscous damping is used to implement the semi-active actuation, and the optimally tuned classical TMD provides the reference response. Keywords: semi-active control, tuned mass damper (TMD), reinforcement learning, damage identification Affiliations:
Jedlińska A. | - | IPPT PAN | Pisarski D. | - | IPPT PAN | Mikułowski G. | - | IPPT PAN | Błachowski B. | - | IPPT PAN | Jankowski Ł. | - | IPPT PAN |
| 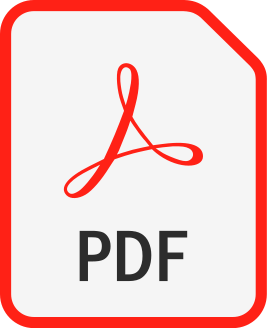 |
3. |
Jedlińska A., Pisarski D., Mikułowski G., Błachowski B., Jankowski Ł., Semi-Active Control of a Shear Building based on Reinforcement Learning: Robustness to measurement noise and model error,
FedCSIS 2023, 18th Conference on Computer Science and Intelligence Systems, 2023-09-17/09-20, Warsaw (PL), DOI: 10.15439/2023F8946, pp.1001-1004, 2023 Abstract: This paper considers structural control by reinforcement learning. The aim is to mitigate vibrations of a shear building subjected to an earthquake-like excitation and fitted with a semi-active tuned mass damper (TMD). The control force is coupled with the structural response, making the problem intrinsically nonlinear and challenging to solve using classical methods. Structural control by reinforcement learning has not been extensively explored yet. Here, Deep-Q-Learning is used, which appriximates the Q-function with a neural network and optimizes initially random control sequences through interaction with the controlled system. For safety reasons, training must be performed using an inevitably inexact numerical model instead of the real structure. It is thus crucial to assess the robustness of the control with respect to measurement noise and model errors. It is verified to significantly outperform an optimally tuned conventional TMD, and the key outcome is the high robustness to measurement noise and model error. Keywords: structural control, semi-active control, reinforcement learning, tuned mass damper (TMD) Affiliations:
Jedlińska A. | - | IPPT PAN | Pisarski D. | - | IPPT PAN | Mikułowski G. | - | IPPT PAN | Błachowski B. | - | IPPT PAN | Jankowski Ł. | - | IPPT PAN |
| 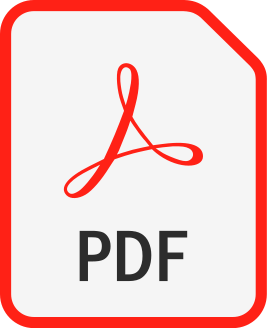 |
4. |
Jedlińska A., Pisarski D., Mikułowski G., Błachowski B., Jankowski Ł., Semi-active structural control using viscous dampers and reinforcement learning,
SMART 2023, 10th ECCOMAS Thematic Conference on Smart Structures and Materials, 2023-07-03/07-05, Patras (GR), pp.589-596, 2023 Abstract: This contribution presents an approach to structural control based on reinforcement learning. Reinforcement learning, a rapidly developing branch of machine learning, is based on the paradigm of learning through interaction with the environment. Here, it is applied in the context of semi-active structural control, where the considered actuators take the form of viscous dampers with a controllable level of damping. The control forces are thus coupled with the structural response, and the formulation is intrinsically nonlinear. The related optimum control problems are usually more difficult than in the case of active structural control systems, which generate and apply arbitrary external control forces. Analytical derivation of the optimum semi-active control is thus rarely possible, so that many control algorithms applied in practice are suboptimal and/or heuristic in nature. Here, an effective control strategy is developed by means of the Q-learning approach. The control algorithm is determined in interaction with the controlled system, that is, by applying initially random control sequences in order to observe, process, and optimize their effects. Such an approach seems to be new and relatively unexplored in the field of structural control. Verification is performed in a numerical experiment, where the Q-learning procedures interact with an independently simulated finite element model of a structure equipped with a tuned mass damper (TMD) and a controllable viscous damper. The results attest to a performance significantly better than that of an optimally tuned conventional TMD. Keywords: Reinforcement Learning, Semi-active control, Structural control, Damping, Vibration Affiliations:
Jedlińska A. | - | IPPT PAN | Pisarski D. | - | IPPT PAN | Mikułowski G. | - | IPPT PAN | Błachowski B. | - | IPPT PAN | Jankowski Ł. | - | IPPT PAN |
| 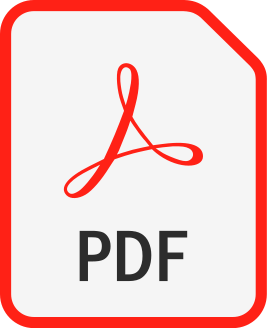 |
5. |
Ostrowski M., Jedlińska A., Popławski B., Błachowski B., Mikułowski G., Pisarski D., Jankowski Ł., Semi-active sliding-mode control for local mitigation of structural vibrations by means of on/off nodes,
SMART 2023, 10th ECCOMAS Thematic Conference on Smart Structures and Materials, 2023-07-03/07-05, Patras (GR), pp.538-548, 2023 Abstract: This contribution presents a sliding-mode control approach for the mitigation of vibrations in frame-like structures. The control is implemented in a semi-active manner, that is, without significant external control forces and substantial power consumption, which are typical for active control approaches. Here, the control is achieved through dynamic, lowcost modification of properties at selected structural nodes. The employed actuators have the untypical form of two-state hinges, which can switch between two extreme states: no transfer of bending moments (effectively a hinge) and full transfer of bending moments (a locked hinge or a typical frame node). Consequently, the control forces are dissipative and coupled to the response. Previous research in this area focused on purely energetic considerations, aiming for global damping of vibrations. In contrast, this paper formulates the control objective in terms of local displacements of a selected degree of freedom, which can be interpreted as the task of isolating it from external excitations. This formulation is employed to define the target sliding hyperplane. The state of the actuators is chosen such that the effective control forces push the structural state toward the target hyperplane. The approach is verified in a numerical example of a six-story shear-type structure subjected to random seismic excitation. Keywords: Structural control, Semi-active control, Sliding mode control, On/off nodes Affiliations:
Ostrowski M. | - | IPPT PAN | Jedlińska A. | - | IPPT PAN | Popławski B. | - | IPPT PAN | Błachowski B. | - | IPPT PAN | Mikułowski G. | - | IPPT PAN | Pisarski D. | - | IPPT PAN | Jankowski Ł. | - | IPPT PAN |
| 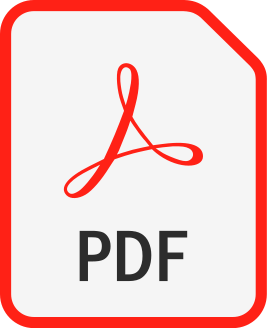 |